by Satalia Team
18 February, 2025
The “now and next” of enterprise AI — what you need to know
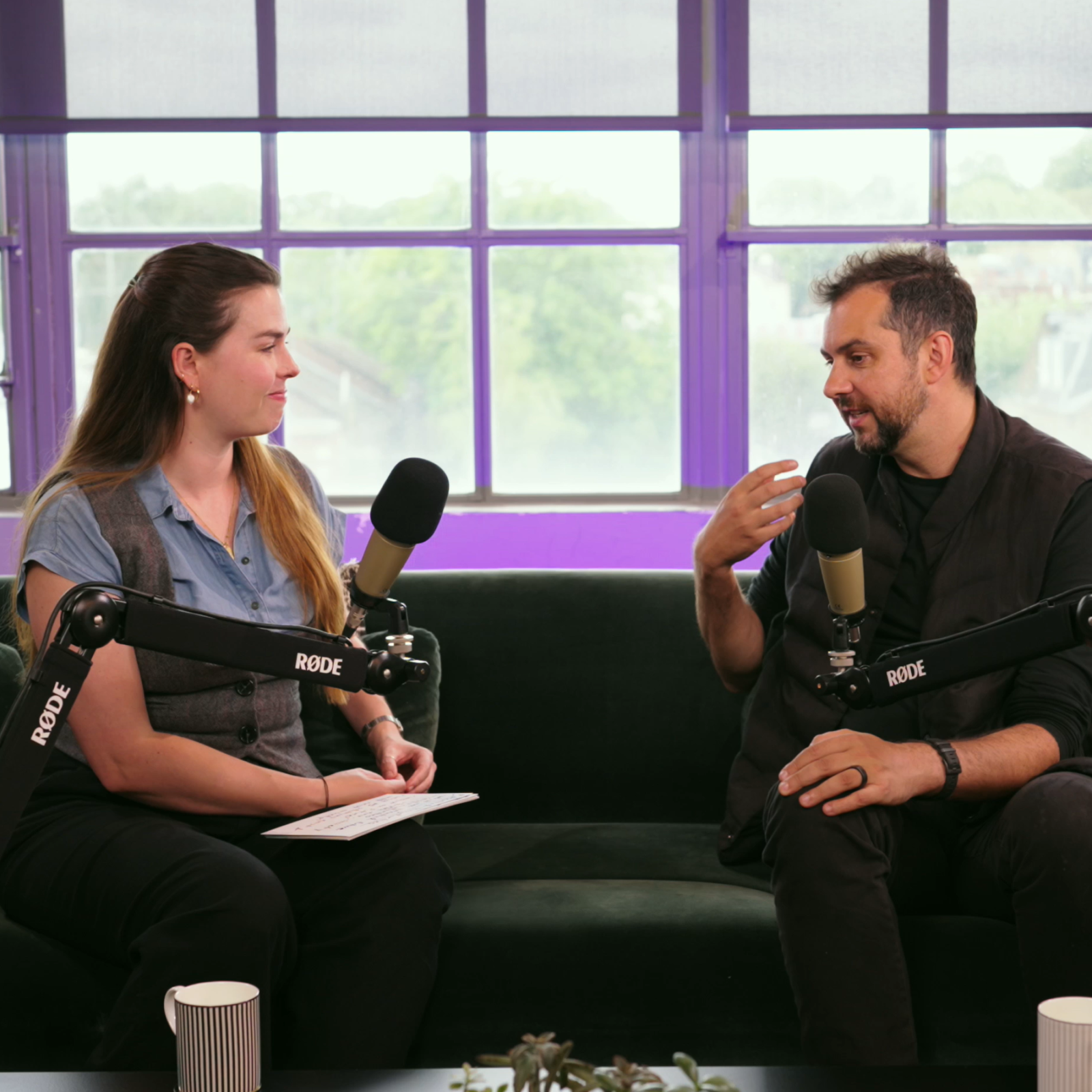
AI is revolutionising industries today — and it’s set to completely reshape our tomorrow…
AIs that market to other AIs. Brain-like AIs 100x faster than ChatGPT. The birth of purpose-driven enterprise — and the end of work as we know it. Some of it’s already here…the rest of it’s on the way, thanks to AI technology.
Satalia CEO & WPP Chief AI Officer Daniel Hulme joined science and tech communicator Maren Hunsberger in a thought-provoking discussion about how artificial intelligence is currently transforming business — and how it could shape the future. Here’s what they discussed…
MH: Where in day-to-day business do we see AI right now?
DH: I guess there are three aspects of an organisation where you can apply AI. Any friction that exists within a company, you can apply technology to alleviate. There are broadly three categories. One is core productivity. Think about writing emails better, creating PowerPoints faster. As far as I’m concerned, everybody will benefit from those improvements in the application of AI.
The second is your supply chain—the flow of goods across your organisation—and where AI can be used to differentiate your business from the next.
The third question, particularly in marketing, media, and communications, is how AI can completely disrupt the industry. You don’t just want to use it to accelerate your digital transformation; you need to ask, “How does it disrupt your business and industry?” Those are the three big questions we apply ourselves to.
MH: Where do you see AI being able to cause that disruption? What does that look like?
DH: I think there are some industries that are relatively immune to the disruption of AI, particularly industries that need to store things or move things around in the physical world. Generative AI, which is one type of AI, is not particularly good at improving those aspects of a supply chain. Typically, algorithms can improve performance by 5, 10, or 15%, whereas some industries, like media, marketing, and communications—where creating content now can be done 10 or 100 times faster — see the creation of content and the ability to test that content against audiences as a real massive disruption.
MH: What does that look like on an organisational level?
DH: It actually takes some time for organisations to adopt technologies. Unfortunately, we go through cycles where we get excited about new emerging technologies — now it’s generative AI — and then people apply those technologies to solving the wrong problems. What tends to happen is there’s a business case that’s created, which takes some time. Then there’s the forming of the project and the building of a solution, which typically doesn’t work. Then they realise they’ve made a mistake and go to vendors that really know what they’re talking about. That takes several years of cycles.
So, I think we’re going through that cycle now, where people are applying generative AI to solving problems that aren’t necessarily ripe for these technologies. Over the next three to five years, we’re going to see real impact in terms of how organisations operate, in terms of efficiency, but also effectiveness. We’re going to be more energy-efficient, faster at creating and disseminating our goods, and it’s also changing the way organisations operate, which I’m very excited about.
MH: How can businesses prepare themselves for that change?
DH: Obviously, there’s been a lot of excitement around data over the past 10 years. Controversially, I would argue that companies have been trying to build data lakes, put some sort of analytics layer on top, and hoped that by extracting insights from data and giving those insights to human beings, it would lead to better decisions. If I’m being honest, giving human beings better insights doesn’t typically lead to better decisions.
I’m a big fan of identifying what is the friction, which is usually some sort of decision-making problem that needs to be solved, and then working backwards. I think two things need to be solved. One is getting your data in the right shape. The other is creating a backlog of frictions that exist across your organisation and then figuring out how to apply the right technologies to solve those frictions.
Actually, I would argue that technology isn’t necessarily the differentiator for organisations. There are three differentiators. One is data, because it’s data that makes the AI smart. The second is AI talent. A lot of organisations think they can attract and retain AI talent, but the reality is they’ll join and leave after a few years. Being able to create an organisation that harnesses bleeding-edge AI talent and applies that to building disruptive solutions is challenging.
The third differentiator is leadership. If leadership isn’t bought into the transformational power of AI, or if they aren’t savvy about applying the right technologies to the right problems, you see a massive amount of misinvestment. One of the things we’ve been doing in Satalia for almost two decades is helping organisations go on that journey of building AI across their supply chain and essentially future-proofing their business.
MH: What kinds of projects has Satalia been a part of that have helped businesses prepare for this future?
DH: One of my favourite clients is DFS, a company here in the UK that manufactures and distributes sofas and furniture. Over the past 10 or so years, we’ve been solving problems across their supply chain. We built their last-mile delivery solution for delivering sofas across the UK. We’ve solved middle-mile challenges, predicted footfall in their stores, allocated staff in their stores, and predicted supplier defaults — whether suppliers are going to default on their supply.
By solving these frictions, we’ve driven a massive amount of value across DFS. What’s also allowed them to do is start to tie these solutions together to create what is called a digital twin. A digital twin is essentially a simulation of your entire organisation. What’s been exciting about the journey with DFS is that they’ve not only applied these innovations to improve their own efficiencies but have also now platformed those innovations.
They’ve tried to become, or are becoming, the Ocado for furniture delivery. They now make this technology available to third parties to do sofa delivery, which allows them to turn their innovations not into a cost centre but into a revenue centre.
MH: So we’re seeing it as a ripple effect across sectors and industries?
DH: Yes, I think the question is, how can you not just use these technologies to solve a problem, but how do you “platformise” them to turn them into revenue generators? Aside from the revenue generation, it also gives you access to data and more talent.
When we say AI, a lot of people may picture a computer or a line of code, whereas the human element is still so essential. Where does AI interact with human decision-making?
There are multiple intersections. The first is the intended use of the technologies. The difference between human beings and AIs is that AIs don’t have intent. Human beings have intent, and it’s the human being that needs to understand whether the intended use of these technologies is ethically appropriate.
The next challenge is how to ask the right questions and get an understanding of the full shape of the problem, which is where consulting comes in. Then you need to build a solution, and often, we’re building solutions that have never been built before. You need to unlock the creative capacity of your workforce to come up with new ideas to solve problems.
MH: How can AI help humans approach these incredibly complex decisions within their organisation?
DH: When it comes to complexity, we need to understand what we mean by complexity. Is it trying to understand an insight — why a particular behaviour is happening? Sometimes, this requires domain knowledge. A bad example I like to use is, if I show you an ad with a black cat, I can use machine learning to predict the clicks, likes, and sales. But what machine learning can’t do is say, “Daniel, if you change that from a black cat to a ginger cat, you’re going to get more clicks, likes, and sales because that person likes Garfield.” AI can help you understand and identify insights that human beings typically haven’t been able to.
You don’t solve those problems with generative AI or machine learning. You solve those problems with optimisation algorithms. Optimisation algorithms, if you’re old enough, used to be called operations research. At Satalia, we’re very fortunate to have a mix of different types of experts in algorithms. What we’re really good at is understanding the nature of the problem and then applying the right algorithms to solve it.
MH: In the example of an organisation that has all these people to match to all these jobs, where does that algorithmic expertise come in?
DH: In that case, it would be the planners. The planners understand the constraints. For example, you don’t work on your birthday, or a person with a certain skill level isn’t allowed to work on a particular client. They build the constraints. The challenge is getting the data to satisfy those constraints.
But there are always things the AI won’t know. For example, you’re not allowed to store information about religious beliefs or whether people are married. In some workforce allocation projects we’ve done before, planners still have to spot whether, for instance, people with a certain religious belief are working on a client they shouldn’t be. There’s always room for human beings in the loop. It elevates humans from trying to solve this complex maths problem to running multiple scenarios. Then the business can decide which scenario suits their needs.
MH: How does Satalia bring that sort of safeguarding to client work?
DH: We have frameworks we use for building safe and responsible AI. We try to make sure the algorithms we use are explainable. The real difference between software and AI is that AI isn’t necessarily very explainable in terms of how it makes decisions. Building explainable algorithms allows you to understand how decisions are made, mitigating bias, and so on.
In the world of WPP, because we can now create content rapidly, we also need a mechanism to test that content. We’re building mechanisms — called brains — to look at content across our supply chain, ensuring it’s not infringing on ad claims, compliance, or upsetting any cultures or minority groups. We can use AI to determine whether content is safe and responsible.
MH: When it comes to content generated by AI, what is Satalia doing to prevent things like misinformation or disinformation?
DH: There are already mechanisms in media marketing and communications, established long before AI, to mitigate these risks. What AI has enabled is doing this exponentially faster. Those frameworks and guardrails are still in place.
What we’re doing is building tools to surface any challenges early on, ensuring we catch them before they become a problem. Over the past two decades, we’ve pioneered frameworks and mechanisms to ask the right questions of the right technologies. The questions you ask when implementing generative AI are different from those for machine learning or optimisation. We’ve built frameworks to navigate this complex world of safety, security, ethics, and governance.
MH: You bring that to every client project, depending on their sector?
DH: Absolutely. It’s something we’re passionate about. What’s really interesting about AI is that I’d argue there are three key questions you need to ask when implementing AI safely.
The first is: Is the intent appropriate? That’s an ethical question. I know lots of people have rebranded themselves as AI ethicists, but I’d argue there’s no such thing as AI ethics. Ethics is the study of right and wrong. AIs don’t have intent — human beings do. The first step is scrutinising intent from an ethical perspective. Once it passes that test, there are two safety questions to address:
Are my algorithms explainable? Can I understand how decisions are made? This isn’t just about ticking boxes for regulations; it’s also about surfacing insights to make better decisions.
Then, what happens if AI goes very right? What happens if AI overachieves its goal? There have been many scenarios in the past where we’ve built solutions for organisations that overachieved their objectives. Fortunately, before they went into production, we identified issues that could have caused problems elsewhere in the supply chain.
For example, with workforce allocation for one of our clients, a large audit firm, we built an AI solution to allocate 5,000 auditors to demand. The KPI for success was a 2% increase in utilisation (billable hours), which would have paid for itself and been a remarkable success. We actually built an algorithm that improved utilisation by 12%, which could have unlocked hundreds of millions of dollars in opportunity. However, other KPIs would have been negatively affected — clients wouldn’t have continuity, employees would have to drive longer distances, and they wouldn’t have time to train. Overachieving on one KPI could have caused adverse effects on others.
Now, we ensure we look across the entire supply chain to build solutions that elevate KPIs positively across the board.
MH: That’s a more nuanced problem with multiple streams of optimisation?
DH: Indeed. It requires human beings to ask the right questions. In the world of marketing, there’s an interesting human bias called “homo-phenotypism.” We, as human beings, tend to resonate or trust people who look and sound like us. If you let AI optimise itself or ads without constraints, it could create a world where you’re essentially selling to yourself. This could enforce bigotry, biases, and social bubbles. We need to consider what happens if AI goes very right and the harm it could cause. These are the things we ask ourselves every day.
MH: It’s an integrated, holistic, wide-view approach?
DH: Exactly. That brings us to the question: We call it artificial intelligence, but what does intelligence mean? What kind of intelligence does AI have, and is it truly intelligence in the sense we understand?
There are lots of popular definitions of AI. The most popular one, and the weakest, is getting computers to do things that humans can do. Over the past three years, we’ve gotten machines to recognise objects in images or converse in natural language like ChatGPT. When machines behave like humans, we assume that’s intelligence, as humans are the most intelligent things we know. But benchmarking machines against humans is a silly thing to do.
Humans are good at finding patterns in about four dimensions and solving problems with up to seven moving parts. Computers can find patterns in thousands of dimensions and solve problems with thousands of moving parts. If I build a machine, give it data, and it makes the same decision tomorrow with the same data, I have automation. Automation is amazing — it allows computers to do things better than humans. But the definition of stupidity is doing the same thing over and over again and expecting a different result.
A really elegant definition of intelligence is “goal-directed adaptive behaviour.” Goal-directed means trying to optimise objectives, like routing vehicles for deliveries, allocating workforces for utilisation, or spending marketing budgets to maximise ROI. Behaviour is how quickly you can answer that question. If you choose the wrong algorithm, it might take longer than the age of the universe to solve even simple problems. If you choose the right algorithm, it differentiates your business.
The key word in this definition is adaptive. Adaptive systems make decisions, learn whether those decisions are good or bad, and adapt to make better decisions next time. If I’m honest, adaptive systems are extremely rare in production because building them is incredibly hard. Satalia is one of very few companies in the world that knows how to build scalable, safe, adaptive systems.
Looking at AI through definitions or technologies isn’t very useful. Over the past several years, we’ve started looking at AI through its applications. I believe there are six categories or families of AI applications that can be applied to any friction across any supply chain in any industry.
MH: There are six categories of AI applications?
DH: Yes. The first is task automation. While task automation isn’t necessarily AI, applying simple algorithms to replace repetitive, mundane, structured tasks can drive huge value for a business. You don’t need shiny technologies like generative AI for this.
The second is content generation—ads, promotional materials, or policies. Generative AI lets us create content beyond text and images, including video and sound. The battleground for organisations isn’t creating generic content, which large language models are good at. It’s creating brand-specific, production-grade, differentiated content. At WPP, we build “brand brains”—models and technologies that let us produce content that’s 95% ready to go out, bringing processes that used to take weeks down to days.
The third category is human representation. Previously, we talked about replacing people in call centres with AIs that sound and look like humans. Now, AI allows us to create “audience brains,” which represent how people perceive and think about content. This helps predict activation—whether a piece of content will lead to more clicks, likes, and sales.
The fourth category is insight extraction. This is what people referred to as AI before generative AI—machine learning to extract insights and make predictions. However, giving humans better insights doesn’t always lead to better decisions. The power of machine learning lies in explaining predictions, e.g., changing an ad’s content to improve performance.
The fifth category is complex decision-making. Many organisational problems are optimization problems. This isn’t about generative AI or machine learning—it’s a different field of computer science. I’d argue solving complex decision-making problems first provides the biggest bang for your buck.
The final category is human augmentation. A few years ago, we’d talk about exoskeletons or cybernetics to make us faster, better, and stronger. Now, we’re building “brains” that represent or augment you. For example, a model trained on your digital footprint—email, calendar, buying habits—can augment decisions like whether to buy a chocolate bar. These digital selves can also be used for workforce allocation, asking questions like, “If I put this person on this team, will they thrive?” It may sound creepy, but employees are embracing these ideas because they feel their digital twins represent them better than five numbers in an HR database.
One of the exciting things about marketing is that we’ll need to learn not only how to market to human beings but also to their AI or digital representations. These six categories help navigate the complex world of AI safety, security, ethics, and governance.
MH: Do you think one dimension will have the biggest impact in the future?
DH: I think complex decision-making—removing humans from making complex decisions they shouldn’t be making—is key. For anything above seven moving parts, humans don’t perform well. Task automation is also critical. By augmenting or removing humans from these processes, we can better understand what data and insights lead to better decisions. Insights and predictions alone don’t usually result in better decisions. Solving the decision-making problem first, whether it’s a complex decision or simple task automation, has the biggest impact.
MH: I see that generative AI is having an impact, especially in the short term, while other technologies, like augmentation, gain prominence.
DH: Absolutely. Generative AI isn’t necessarily suited for complex decision-making yet, but I think these technologies will evolve. Currently, generative AI provides generic knowledge, like an intoxicated graduate—it gets things wrong a lot. Over the next six months, we’ll see it graduate to a master’s level of reasoning, and in another 18 months, it’ll reach a PhD level, not just in knowledge but in problem-solving—breaking problems down, creating hypotheses, and running experiments. Within 18 more months, we may even have a “postdoc” level AI, and in another 18 months, we could have a professor in our pocket.
I don’t know how quickly these capabilities will permeate businesses, but by the end of this decade, having a personal “professor” AI could change everything.
MH: What about issues like inaccurate or biased data in large language models? For example, what if it pulls data from sources like Reddit, where trolls post misinformation?
DH: You’re right. Large language models are inherently biased based on the data they’re trained on. The difference between today’s models and the ones we’ll see in a year lies in reasoning. Currently, if the data supports “Socrates is a man” and “All men are mortal,” the model can’t infer that “Socrates is mortal” unless it’s explicitly in the data. Reasoning will allow AI to make these inferences and start checking itself for inaccuracies or statistical errors. Over the next 12 months, we’ll see a step change in AI’s intelligence.
MH: How do we avoid building our biases into machine learning or AI algorithms?
DH: Bias arises from two main sources: data and intent. To avoid bias, you need diverse and varied data. However, too much variation can confuse the system. Intent is equally critical—if your goal is to hire “smiley and happy” people, you’ve already introduced bias. That’s not an AI problem; it’s a human ethics problem.
One way to address bias is through a concept called “agentic computing.” This involves using specialised, biassed AI agents for different domains, each representing specific knowledge or perspectives. By having these agents collaborate and argue, we can achieve better solutions, much like diverse human teams bring varied perspectives to solve problems.
MH: So this approach mirrors how humans address biases by bringing them together to find common solutions?
DH: Exactly. Future AI systems must be adaptive, learning from their actions and improving. Currently, in production, systems are static. You build them, put them in production, gather data, learn from mistakes, rebuild the model, and redeploy it. This process is time-consuming and energy-intensive.
Emerging technologies like neuromorphic computing aim to address this. Large language models mimic how our brains work but are far from it. Our brains operate on the power of a light bulb and learn quickly. For example, you only need to see something once or twice to recognize it. Neuromorphic computing, inspired by how our brains spike and activate only relevant portions, is more energy-efficient and adaptive.
Neuromorphic computing could disrupt industries by enabling faster, more efficient systems, especially in the physical world, like drones and robotics. At Satalia, we’re exploring these technologies and their potential to unlock the next technological revolution.
MH: As businesses approach this AI-driven future, what can they do to prepare for technologies like neuromorphic computing?
DH: Businesses involved in physical operations—moving goods or engaging with the physical world—should start exploring these technologies. Neuromorphic computing helps us understand and influence perception. It’s also worth engaging with experts or companies like WPP to understand how AI can future-proof your business.
MH: Are there examples of how Satalia is preparing its clients for the future?
DH: We’re building AI infrastructures designed for adaptability. Solutions are architected not only for their immediate purpose but also to be reusable across supply chains. This ensures they’re ready for replacement by smarter, more capable AI as it emerges.
One example is using neuromorphic technology to analyse large amounts of video. It can identify patterns and similarities quickly, such as recognizing that scenes from different Disney movies share the same animation skeleton. These technologies are not only faster but also far more energy-efficient, consuming significantly less power than traditional systems.
Neuromorphic AI is both energy- and learning-efficient, mimicking how human brains work. Instead of propagating data across an entire system, it activates only small portions, making it 10 to 100 times more energy-efficient than large language models.
MH: What are some potential singularities you envision for AI?
DH: The concept of a singularity comes from physics, describing a point beyond which we can’t see. In AI, the technological singularity refers to building a brain a million times smarter than us. Some experts believed this might happen in 30—40 years, but now we think it could occur in the next 10—20 years.
At least six singularities need our attention:
- Political Singularity: AI-driven misinformation, bots, and deepfakes challenge political systems and can be exploited by bad actors. However, we can mitigate these risks with the right safeguards.
- Environmental Singularity: Consumption pressures planetary boundaries, but AI can help. Optimising supply chains can halve the energy needed to run the planet. For example, solving delivery and workforce allocation problems has reduced carbon emissions by 20—25%. Optimising across supply chains could amplify these benefits.
- Social Singularity: Advancements in AI and medicine mean some people alive today might not have to die. AI can monitor and maintain human health like a car getting regular tune-ups, preventing breakdowns.
- Technological Singularity: We may soon become the second most intelligent species on the planet. Companies like Satalia are working on safe, aligned AI to ensure this intelligence benefits humanity.
- Legal Singularity: Surveillance could become ubiquitous. AI can understand and change perceptions, making it powerful but potentially dangerous if misused by bad actors. Regulation and ethical use are critical to avoiding misuse.
- Economic Singularity: AI can eliminate friction in producing and delivering goods like food, healthcare, and education, potentially making them nearly free. This could lead to a world where everyone has access to what they need to thrive.
MH: What would people do in a world where they don’t need paid work?
DH: When asked, most people initially say they’d play golf, travel, or spend time with family and hobbies. But when pushed further, they admit they’d want to contribute to humanity. AI can help remove economic constraints, empowering people to use their creativity for the greater good.
In this world, traditional businesses with profit motives might no longer exist. Instead, people could create platforms and solutions for social good without worrying about financial models. For example, connecting isolated elderly people with communities that want to learn from them could be meaningful, even if it isn’t economically viable today.
This vision isn’t utopia—it’s protopia: a world continuously improving as more people are freed from economic constraints and empowered to contribute.
MH: How can businesses today bridge the gap to this protopia future?
DH: Focusing on purpose is critical. Businesses without purpose won’t attract customers or talent, especially AI talent. People want to work on meaningful projects that improve lives. By identifying and solving organisational frictions with AI, businesses can build adaptable infrastructures that make goods and services more accessible while remaining relevant.
For example, organisations can use AI to reduce costs, increase efficiency, and expand their reach, all while keeping a balance between profitability and purpose. Leadership is key—understanding the transformational power of AI ensures investments are made in opportunities that truly differentiate the business.
MH: What are examples of how Satalia helps businesses with AI?
DH: Satalia focuses on building AI infrastructures that are adaptable and reusable across supply chains. Solutions are designed to evolve as new technologies emerge, ensuring long-term value.
One current project involves ingesting massive amounts of video data to identify patterns quickly and efficiently.
Disney has reused the same kind of scene across movies—for example, using a scene from Mowgli in place of Cinderella. While these scenes look different to humans, they are strikingly similar. Neuromorphic technologies help identify such similarities in content and do so in an incredibly energy-efficient way.
MH: What do we mean by energy-efficient AI?
DH: Your brain operates on the power of a light bulb and learns very quickly. You don’t need to be shown something more than once or twice to understand it. In contrast, large language models consume vast amounts of energy to learn and adapt. Our brains spike, activating only small portions at a time rather than propagating signals throughout the entire brain. Neuromorphic technologies mimic this process—they are based on how our brains work.
MH: So when we say “energy-efficient AI,” we mean reducing the amount of energy drawn from the power grid for both learning and operation?
DH: Yes. Academic studies have shown these technologies to be 10 to 100 times faster and more energy-efficient than large language models.
MH: What are some of the potential future singularities you envision for AI?
DH: The term “singularity” comes from physics—a point in time we can’t see beyond. It was adopted by the AI community to describe the “technological singularity,” a moment when we create a brain far more intelligent than humans. Initially thought to be 30—40 years away, some believe it could happen in the next 10—20 years. For example, a startup recently raised a billion dollars with a valuation of $5 billion despite having only five employees.
There are at least six singularities we need to address, which I frame using the PESTLE framework:
- Political Singularity: AI misinformation, bots, and deepfakes have already challenged political systems and will continue to do so. We must mitigate the risks of bad actors cloning people or committing fraud using these technologies. I believe this is a solvable problem.
- Environmental Singularity: Consumption gives people access to goods and services but puts pressure on our planet’s resources. By applying AI correctly, we can cut the energy needed to run the planet by at least half. For example, solving Tesco’s last-mile delivery problem reduced carbon emissions by 20—25%. Optimising supply chains further can reduce emissions and unlock greater capacity.
- Social Singularity: Some scientists believe people alive today might not have to die. AI is advancing medicine, enabling us to monitor and “clean out” our bodies. Like a car that never breaks down with proper maintenance, humans could achieve similar longevity.
- Technological Singularity: We may become the second most intelligent species on Earth within the next decade. This is why companies like Satalia and WPP are investing in building safe, aligned AI. Neuromorphic computing and other technologies could allow us to create AI beneficial to humanity.
- Legal Singularity: When surveillance becomes ubiquitous, AI could understand and influence human perception. This poses a powerful risk if used by bad actors to accumulate wealth and power. The marketing industry and regulators will play critical roles in mitigating this danger.
- Economic Singularity: Over 17 years, Satalia has built AI solutions that enable people to do more purposeful work without losing their jobs. In the next decade, we’ll see an explosion of innovation and opportunity. AI can be a source of energy to drive humanity forward. However, rapid technological job displacement could disrupt economies, leading to social unrest. Mechanisms like a four-day workweek and universal basic income are being explored to manage this risk.
On the other hand, AI could remove friction from the creation and dissemination of goods like food, healthcare, education, and energy, reducing costs to nearly zero. Imagine a world where everyone has access to the essentials to survive and thrive. When asked what they would do without a paid job, most people say they would create, travel, spend time with loved ones, or contribute to humanity. I believe humans have an innate desire to make the world better. Economic constraints often prevent this, forcing people to live only for themselves.
This belief is why I joined WPP. Its purpose—to use the power of creativity to make a better future—aligns with Satalia’s vision of a world where everyone is free to live beyond themselves. By applying AI the right way and helping clients achieve their purpose, we can create futures that benefit all of us.
MH: What would businesses look like in a world where goods and services are free?
DH: Traditional businesses, with shareholders and a focus on ROI, might no longer exist. Instead, individuals with resources could engage with AI to create platforms connecting people. For example, someone might link isolated older people with communities eager to learn, creating meaningful experiences. These efforts wouldn’t rely on economic models but on the ability to build platforms for free, empowering creativity and improving the world.
MH: How can businesses today, still profit-driven, prepare for this future?
DH: Any friction within an organisation can likely be addressed with AI. However, it’s essential to have a purpose. Without purpose, businesses won’t attract customers or the AI talent they need. Employees, too, want to work on meaningful projects that improve lives. Leaders must understand AI’s transformational potential and invest wisely in opportunities that differentiate their businesses.
Speak to an expert Satalia advisor about how AI can transform your business.
Stay in the know
Join our community now for the latest industry news, trends, valuable insights, and updates on our products and services.