by Satalia Team
4 March, 2025
How will AI help businesses with complex decision-making in the future?
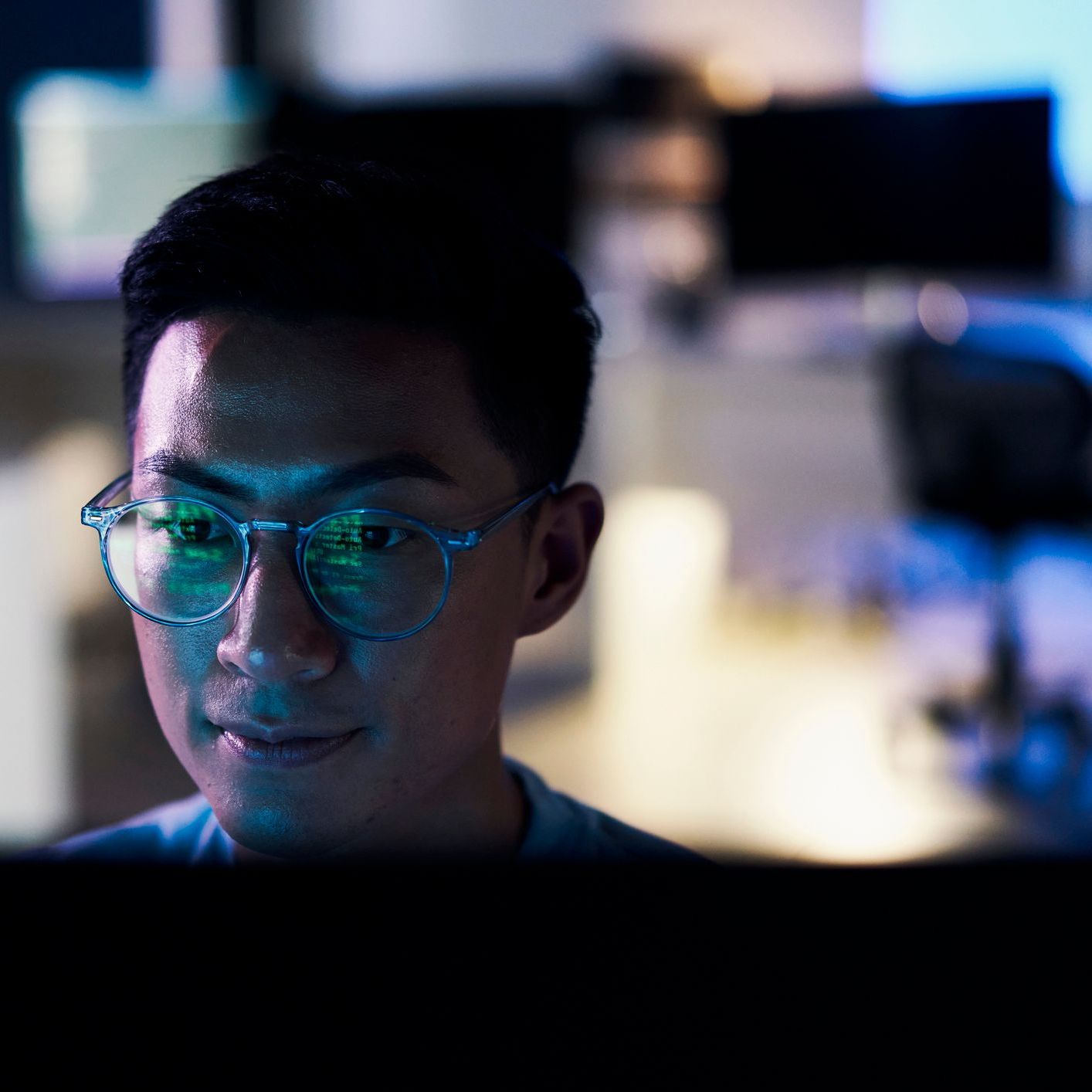
How can businesses benefit from AI-powered decision-making, today and tomorrow?
AI is reshaping how businesses tackle complex decision-making, helping us do more, do it faster, and get better outcomes. Today, AI can already handle problems with thousands of moving parts that are way too intricate for human minds.
But should we trust it to make decisions on our behalf? Can the current generation of generative tools, like ChatGPT, be let loose on businesses’ biggest challenges? And how can businesses explain AI-powered decision-making to customers and clients if the AI can’t explain how it made those decisions? Read on to find out…
Ever-increasing complexity in business will require more insightful AI solutions
When we’re talking about complexity, it’s essential to know exactly what we mean. In AI terms, it’s often about using technology to help us understand why a trend emerges, or why a particular behaviour happens. This is where both the current generation of generative AI technology, and our own human minds, tend to struggle…
Imagine you run an ad featuring a black cat. A campaign dashboard, driven by generative AI, tells you how many clicks, likes, or sales that ad generated. But it can’t tell you why changing that black cat to a ginger cat might get you better results. That’s where emerging AI tech is beginning to help us uncover insights that have previously been hidden to human minds. It can spot the reason for the person’s behaviour; the user clicked the ad with the ginger cat because they love Garfield. There’s far too much complexity for a human to spot that correlation.
And while the current crop of generative AI and machine learning systems might be great for generating insights, they’re not the solution for this type of problem.
What if we had AI that worked more like human brains?
Neuromorphic computing is a new and exciting way to design computer systems that mimic how the human brain works. Unlike traditional computing, which relies on sequential processing, neuromorphic systems use networks of artificial neurons that communicate with each other, similar to how our brains send signals.
We’re just scratching the surface when it comes to neuromorphic computing, but it could be game-changing for industries that operate in the physical world—think drones, robotics, and more. Like a human brain, these systems become much more efficient, faster, and smarter over time.
At Satalia, we’re really excited about the potential this has to unlock the next technological revolution, making AI systems that can adapt more intuitively and operate with a fraction of the energy used by current large language models (LLMs) like ChatGPT— whose energy demands are well publicised. It’s one thing to build smart AI, but imagine an AI that can learn in real time, like a human; adapting, learning, and growing more useful by the day, all while using a fraction of the energy and resources of the current generation of AIs.
The implications for neuromorphic computing in business are huge. But it’s important to reiterate that while AIs may get better at mimicking how human brains work, they will never replace the unique intuition humans bring to the table in the decision-making process. Instead, this type of AI will serve to further elevate humans above routine work, while also removing bias from important decisions.
The right solution for the problem at hand
Most organisational problems are optimisation issues. These problems tend to be intricate, often involving thousands of moving parts; the kind of problem-solving humans struggle with. Instead of leaving people to flounder, we’ll increasingly be able to deploy neuromorphic “AI brains” to handle the heavy lifting and streamline the path to the best outcomes.
Meanwhile, optimisation algorithms—what old-schoolers used to call operations research—are better suited to tackling certain complex decisions. They can solve complex problems by systematically evaluating every possible option under multiple constraints. They use mathematical models to explore combinations, identify patterns, and narrow down to the best outcome—handling logistical challenges like scheduling, resource allocation, or routing far more efficiently than machine learning, and far more effectively than humans can.
Will we still need humans in the loop?
Humans bring essential intuitive context that AI can’t. That will never change. But the businesses that will succeed in the future will be the ones that support human intuition AI-driven insights.
For example, let’s say we’re using AI to allocate 60 consultants across 60 client projects. The possible combinations – 60 to the power of 60 – is greater than the number of atoms in the universe. AI is great at crunching all of those combinations. But it can miss context. For example, it can’t store sensitive data about someone’s religious beliefs or their marital status. So there are scenarios a machine alone can’t navigate, like ensuring that an employee with a specific belief system isn’t assigned a client that could present a conflict (like somebody of a particular faith being assigned an alcohol client or gambling client). And that’s where the human element is crucial.
We often think more insights and data mean better decisions, but without the right tools to process and use those insights, humans are often left overwhelmed. Our brains are well-equipped to deal with situations involving up to four variables or factors at the same time, like navigating space (height, width, depth) plus a fourth factor, like time. AI can handle thousands of dimensions simultaneously. Machine learning models and optimisation algorithms excel at analysing vast amounts of data, finding patterns, and solving problems with hundreds or even thousands of interrelated variables. On the other hand, it’s important to remember that there will always be context AI doesn’t understand.
Rather than wasting time manually solving intricate mathematical puzzles, key decision-makers will increasingly use AI to run different scenarios and make an informed choice that truly fits the needs of the business, helping them understand what data leads to better choices, and empowering them to act on that knowledge. AI will increasingly tackle complexity so humans can focus on what truly matters—bringing creativity, judgement, and human intuition to the table.
As AI gets more powerful, explaining its complex problem-solving will become paramount
For AI and optimisation algorithms to really make a difference, they must be safe, transparent, and trustworthy. But they also need to make explainable decisions; i.e. be able to show you how and why recommendations or decisions are being made. Imagine your AI not just making choices but actually explaining the thinking behind them, helping identify and mitigate biases. That empowers the humans in the loop to gain a better understanding of those recommendations and decisions, meaning they can apply their intuition to intervene or augment those decisions where necessary.
The real difference here is between traditional software and AI that can be both powerful and understandable. It’s all about ensuring we’re doing the right thing, not just ticking boxes for compliance but making sure we’re working with tools we can really trust.
What if those decisions are too effective?
It’s natural and diligent to ask “what if the AI goes wrong?” But an equally important question is “what if it goes very right?” What if the AI’s decision-making is so effective, it massively exceeds expectations or way outperforms KPIs?
Take our work with a major audit firm as an example. They needed a solution to allocate 5,000 auditors, aiming for a 2% increase in utilisation. That 2% might not sound like much, but it would have made the project profitable on its own.
When we built the algorithm, it actually boosted utilisation by a massive 12%. That could have unlocked hundreds of millions in revenue opportunities, but we quickly realised that such success came with other consequences—employees had to drive longer distances, clients wouldn’t get consistent service, and there was less time for training. Success in one area meant setbacks in others.
So, we made sure to look at the full picture, balancing performance across all metrics rather than focusing on just one. It’s about creating solutions that spell benefits across the business and its people, not just moving one KPI by such a great margin that it causes damage elsewhere.
In short, the successful businesses of the future will be the ones paying close attention to potential unintended consequences of using AI to solve complex problems.
How do we build AI that can solve the complex problems of the future?
It’s important that AI infrastructures aren’t just built for today, but for the future. It’s not just about solving immediate challenges—it’s about creating systems that can evolve, adapt, and keep on delivering value as new technologies emerge.
Solutions should be designed with adaptability baked in, making sure they’re reusable across supply chains and ready to be replaced by even smarter AI as it develops. This is how we stay ahead—not by standing still but by embracing change, adapting quickly, and always aiming to make complex decision-making better, smoother, and more effective.
Speak to an expert Satalia advisor today about how AI can transform your business.
Stay in the know
Join our community now for the latest industry news, trends, valuable insights, and updates on our products and services.