by Satalia Team
AI thought Leadership
The latest industry-leading AI insights from Satalia
Featured insight
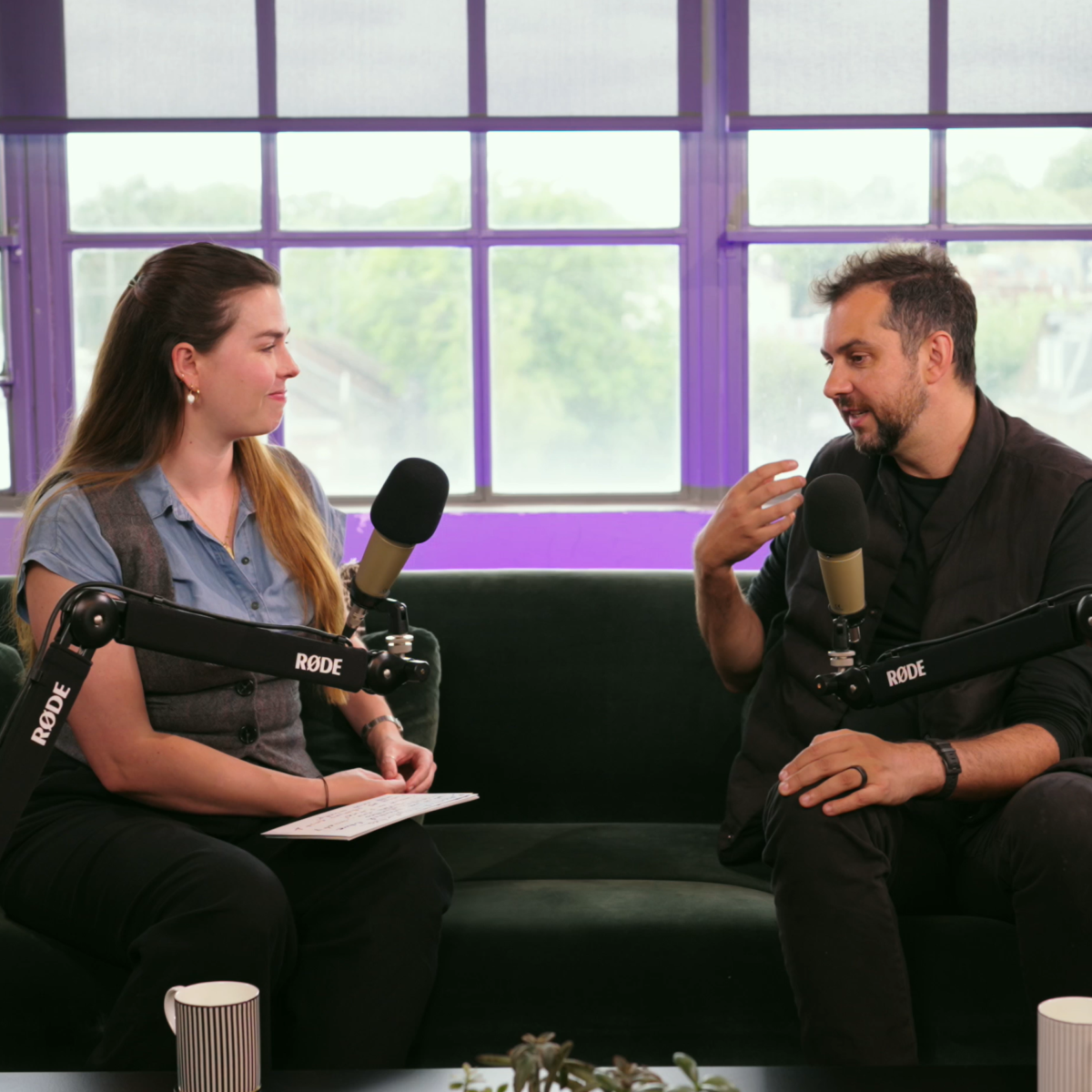
13 June, 2025
AI in marketing – what do businesses need to know?
Marketing is being rebuilt from the ground up by AI. From synthetic focus groups to brand-safe content in seconds, discover how intelligent systems are transforming creativity, performance, and ROI...
Insights
Filter by
Product/service
Stay in the know
Join our community now for the latest industry news, trends, valuable insights, and updates on our products and services.