Our work
We could save you 11 million delivery miles per year
Or deliver a 200x ROI. Or improve efficiency equivalent to adding 140 people – like we’ve done for other clients.
But it’s not just about you. It’s also about your customers.
Because when we create solutions that improve their lives, everyone wins.
It’s how we helped DFS earn an 8% uplift in net promoter score through last-mile delivery transformation. And it’s how we helped Woolworths Australia increase delivery capacity by 30% to get fresh groceries to their customers quicker than ever.
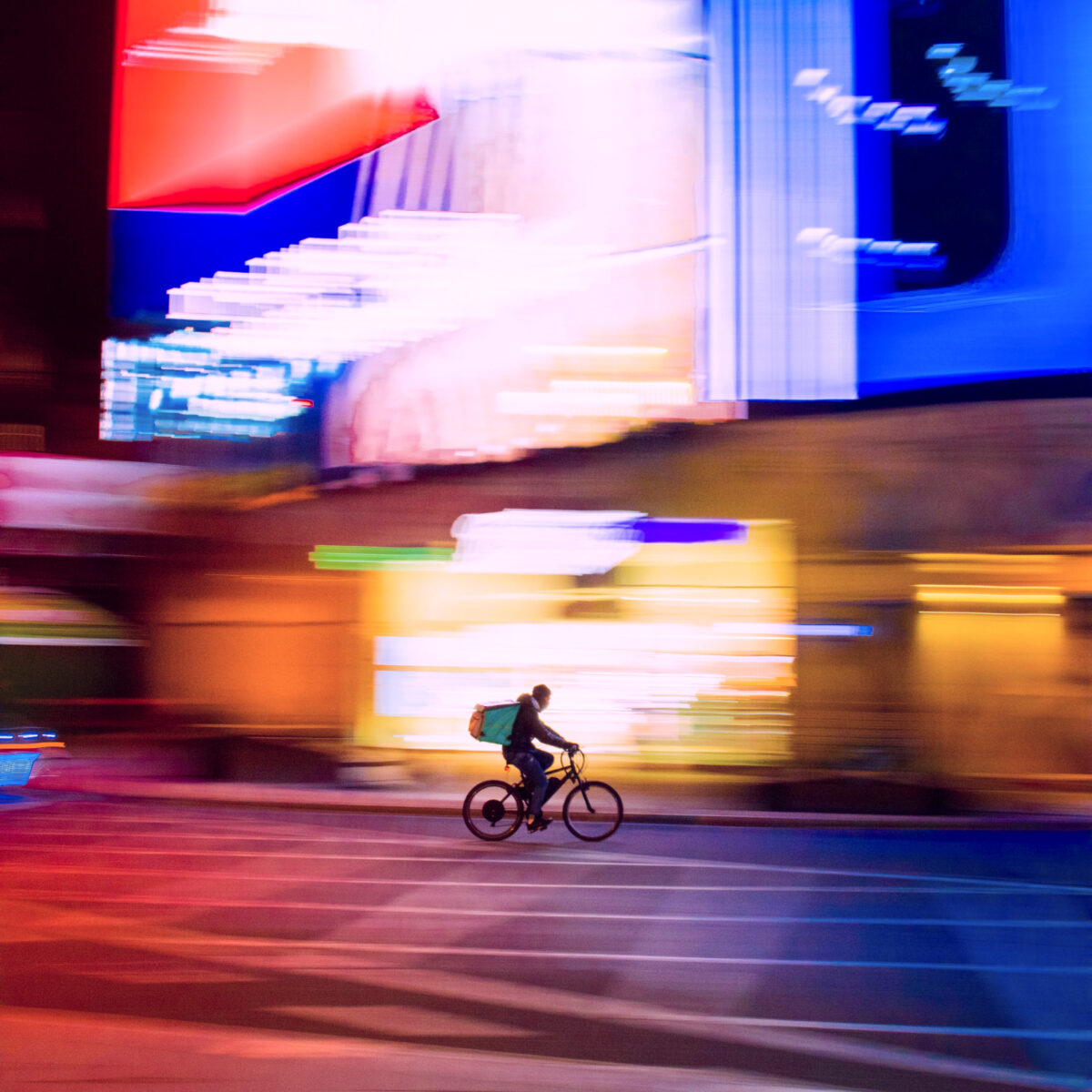
AI for transport, supply chain & logistics
Simulation of last mile capability for large supermarket chain
Satalia helped a large supermarket chain scale their delivery offering by running ‘what if?’ scenarios. These identified changes required to scale their business while maintaining customer experience.
Increase in delivery capacity through simulation experiments